Messi H.J. Lee
Washington University in St. Louis. University City. Missouri.
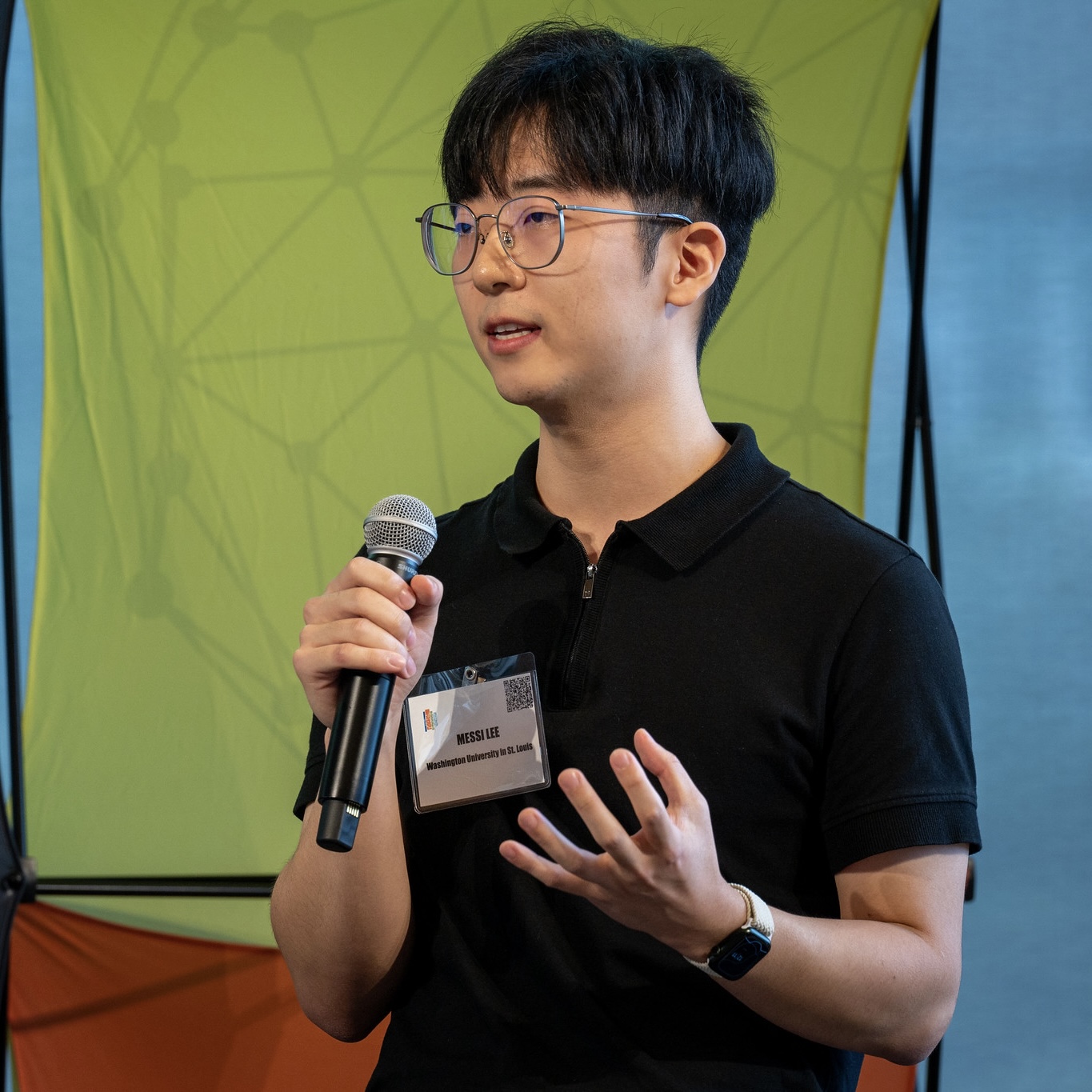
I’m a fifth year PhD Candidate in the Division of Computational and Data Sciences at Washington University in St. Louis (WashU). I am part of the Psychological & Brain Sciences Track, doing research at the Diversity Science Lab. My advisors are Calvin K. Lai in the Department of Psychology at Rutgers University and Jacob M. Montgomery in the Political Sciences Department at WashU.
My research primarily focuses on using text-as-data approaches to measure bias in AI technologies such as Large Language and Multimodal Models. Recently, I published a paper at the Proceedings of the 2024 ACM Conference on Fairness, Accountability, and Transparency, studying homogeneity bias in LLMs where we find that these models portray socially subordinate groups as more homogeneous compared to their dominant group counterparts.
After completing my PhD in May of 2025, I intend to fulfill my military service obligation in South Korea as a Technical Research Personnel (전문연구요원), preferably continuing research in the areas of AI Bias, Computational Social Science, and Natural Language Processing. If there are any relevant opportunities I should know about, please feel free to send me an email at: hojunlee[at]wustl[dot]edu.
news
Oct 11, 2024 | I received a $1,000 Small Grant from the Center for the Study of Race, Ethnicity & Equity to expand my research on homogeneity bias in Large Language Models. This study will explore a broader range of models and parameter settings to examine when the bias appears in model outputs. |
---|---|
Sep 30, 2024 | My submission to the Annual Convention of the Society for Personality and Social Psychology (SPSP) 2025 titled, “More Distinctively Black and Feminine Faces Lead to Increased Stereotyping in Vision-Language Models”, was accepted for poster presentation. |
Jul 11, 2024 | The pre-print of my new paper “Probability of Differentiation Reveals Brittleness of Homogeneity Bias in Large Language Models” was made available on ArXiv. We find that homogeneity bias in Large Language Models, as measured by probability of differentation, is volatile across situation cues and writing prompts. |
Jul 10, 2024 | The preprint of my new paper “More Distinctively Black and Feminine Faces Linked to Increased Stereotyping in Vision-Language Models” is now available on ArXiv. We find that prototypically Black and feminine faces are subject to greater streotyping in Vision Language Models. Look out for my blog post on this article! |
Jul 08, 2024 | I completed my role as Reviewer for the ACL 2024 Student Research Workshop (SRW), where I reviewed quality submissions related to Computational Linguistics, Natural Language Processing, and Bias and Fairness. |